Maximizing Agricultural Efficiency: The Role of Agriculture Datasets for Machine Learning
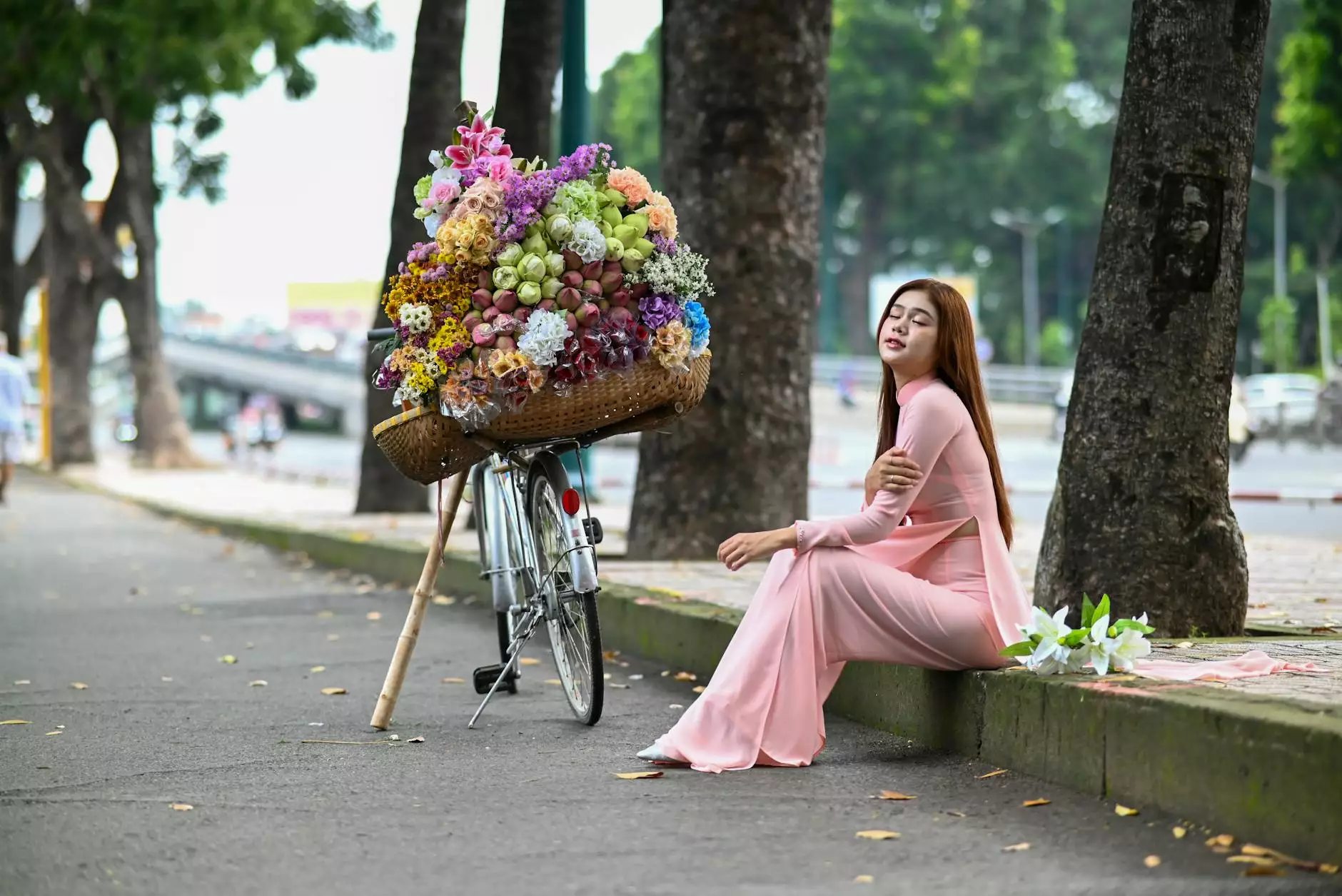
In the ever-evolving landscape of modern agriculture, the integration of machine learning and advanced data analytics is not just a trend but a necessity. The use of agriculture datasets for machine learning is paving the way for innovative practices that enhance productivity, sustainability, and profitability. In this comprehensive article, we will explore the rich realm of machine learning in agriculture, its applications, benefits, and how businesses like Keymakr are positioned to leverage these advancements.
Understanding Agriculture Datasets for Machine Learning
Before diving deeper, it's essential to grasp what agriculture datasets for machine learning entail. These datasets consist of structured and unstructured data collected from various agricultural activities, providing a treasure trove of information ready for analysis. The data can originate from:
- IoT devices monitoring crop health
- Satellite imagery tracking land use and vegetation
- Weather patterns impacting crop growth
- Soil composition and nutrient levels
- Pest and disease incidence reports
By harnessing these datasets, machine learning algorithms can identify patterns, forecast trends, and provide actionable insights to farmers and agribusinesses.
The Significance of Machine Learning in Agriculture
Machine learning offers profound benefits to the agricultural sector, enabling enhanced decision-making and operational efficiency. Here are some key advantages:
1. Precision Agriculture
Precision agriculture focuses on optimizing field-level management regarding crop farming. The use of agriculture datasets allows farmers to apply the right amount of inputs (like water, fertilizers, and pesticides) tailored to specific areas of farmland. This data-driven approach leads to:
- Reduced resource waste
- Increased crop yield
- Enhanced soil health
2. Crop Prediction and Analysis
Accurate crop yield prediction is critical for planning and maximizing profit. Utilizing agriculture datasets for machine learning, farmers can analyze historical data and current conditions to forecast yields, considering factors like:
- Weather conditions
- Market demand
- Soil health metrics
Machine learning models can be trained to predict not just crop yields but also potential pest outbreaks, enabling proactive management.
3. Soil Health Monitoring
The health of the soil is fundamental to successful agriculture. Utilizing machine learning, farmers can analyze soil data to ensure optimal conditions for crops. This includes:
- Identifying nutrient deficiencies
- Assessing moisture levels
- Monitoring erosion and degradation
4. Geographic Information System (GIS) Integration
GIS technology, when combined with machine learning, enables agronomists to visualize and analyze data geographically. This integration helps in:
- Mapping crop productivity
- Identifying optimal planting zones
- Planning irrigation systems
Challenges in Utilizing Agriculture Datasets
While the potential benefits of implementing agriculture datasets for machine learning are compelling, several challenges must be navigated:
1. Data Quality and Availability
The accuracy and completeness of datasets are crucial for machine learning models to function effectively. In agriculture, data may be sparse or inconsistent, leading to unreliable models.
2. Integration of Diverse Data Sources
With various data sources in play – from weather stations to IoT sensors to satellite data – harmonizing this information for machine learning can be complex. It's essential to have robust frameworks in place for data integration.
3. Skills Gap in Agribusiness
Many farmers and agribusiness professionals may lack the technical expertise to implement machine learning solutions. Education and training in data analytics and machine learning are vital for widespread adoption.
Innovative Applications of Machine Learning in Agriculture
The agricultural sector is rife with innovation, and machine learning applications are at the forefront. Here are some notable examples:
1. Automated Irrigation Systems
Advanced irrigation systems utilize machine learning algorithms to predict the optimal watering schedules based on real-time weather data and soil moisture levels, ensuring crops get the right amount of water.
2. Pest Detection and Management
Machine learning models can analyze images from drones or cameras to identify pests' presence early. This enables farmers to take prompt actions, reducing pesticide usage and increasing overall crop health.
3. Supply Chain Optimization
By applying machine learning to agricultural supply chains, businesses can forecast demand more accurately, optimize inventory levels, and improve logistics, ensuring that fresh produce reaches consumers faster.
Future Trends in Agriculture and Machine Learning
The synergy between agriculture and machine learning is expected to grow exponentially. Here are some emerging trends that hold promise for the future:
1. Increased Use of Big Data
With the continuous generation of vast amounts of data, leveraging big data analytics combined with machine learning will revolutionize how we approach farming. This will include enhanced predictive analytics and trend forecasting.
2. Blockchain Technology for Traceability
By integrating blockchain with machine learning, farmers can ensure transparent traceability of produce from farm to table. This application will enhance food safety and quality assurance.
3. Sustainable Farming Practices
Machine learning will play a pivotal role in promoting sustainability, helping farmers make data-driven decisions that minimize environmental impact, conserve water, and reduce chemical inputs.
Getting Started with Agriculture Datasets for Machine Learning
For businesses and professionals looking to harness the power of agricultural datasets for machine learning, here are some initial steps to consider:
- Identify Data Sources: Start by identifying relevant data sources, including public datasets, satellite imagery providers, and local agricultural experts.
- Invest in Tools and Technologies: Utilize machine learning platforms and tools that simplify data analysis and model training.
- Foster Partnerships: Collaborate with tech companies specializing in agri-tech and data analytics to enhance your capabilities.
- Continuous Learning: Keep abreast of the latest advancements in machine learning and agriculture through courses, webinars, and industry conferences.
Conclusion: The Future of Agriculture with Machine Learning
In conclusion, agriculture datasets for machine learning have the potential to transform the agriculture industry by enhancing productivity, promoting sustainability, and driving profitability. As we look to the future, embracing these technologies will be crucial for farmers and agricultural businesses aiming to thrive in a competitive landscape. By leveraging data-driven insights and machine learning innovations, we can ensure a more efficient, sustainable, and prosperous agricultural environment.
For businesses like Keymakr focusing on software development, integrating machine learning solutions within agriculture sector offerings could not only streamline operations but also position them as industry leaders in a rapidly advancing field.
agriculture dataset for machine learning